
How complex is your life? Computer scientists found a way to measure it
Karlo Doroc, The University of Melbourne
Nobel laureate economist Richard Thaler famously quipped:
People aren’t dumb, the world is hard.
Indeed, we routinely encounter problems in our everyday lives that feel complex – from choosing the best electricity plan, to deciding how to effectively spend our money.
Australian pay hundreds of millions of dollars each year to comparison websites and consumer-focused groups such as CHOICE to help them make decisions about products and services.
But how can we objectively measure how “complex” our decisions really are? Our recently published research offers one potential way to do this, by drawing on concepts from computer and systems science.
Why bother measuring complexity?
There are several factors when it comes to measuring complexity in any scenario. For instance, there may be a number of options to choose from and each option may have several different features to consider.
Suppose you want to buy jam. This will be easy if there are only two flavours available, but difficult if there are dozens. Yet choosing an electricity plan would be much harder even with just two options.
In other words, you can’t isolate one particular factor when trying to determine the complexity of something. You have to consider the problem as a whole – and this requires a lot more work.
The ability to accurately measure complexity could have a wide range of practical applications, including informing the design of:
- regulation on how complex products should be
- easy to navigate digital systems including websites, apps and smart device programs
- easy to understand products. These may be financial products (superannuation and insurance plans, credit card schemes), physical products (devices) or virtual products (software)
- artificial intelligence (AI) that offers advice when problems are too complex for humans. For example, a scheduler AI may let you book meetings yourself, before jumping in to suggest optimal meeting times and locations based on your history.
How we study human decision-making
Computer science can help us solve problems: information goes in and one (or more) solutions come out. However, the amount of computation needed for this can vary a lot, depending on the problem.
We and our colleagues used a precise mathematical framework, called “computational complexity theory”, that quantifies how much computation is needed to solve any given problem.
The idea behind it is to measure the amount of computational resources (such as time or memory) a computer algorithm needs when problem-solving. The more time or memory it needs, the more complex the problem is.
Once this is established, problems can be categorised into “classes” based on their complexity.
In our work, we were particularly interested in how complexity (as determined through computational complexity theory) corresponds with the actual amount of effort people must put into solving certain problems.
We wanted to know whether computational complexity theory could accurately predict how much humans would struggle in a certain situation and how accurate their problem-solving would be.
Testing our hypothesis
We focused on three types of experimental tasks, for which you can see examples below. All of these task types sit within a broader class of complex problems called “NP-complete” problems.
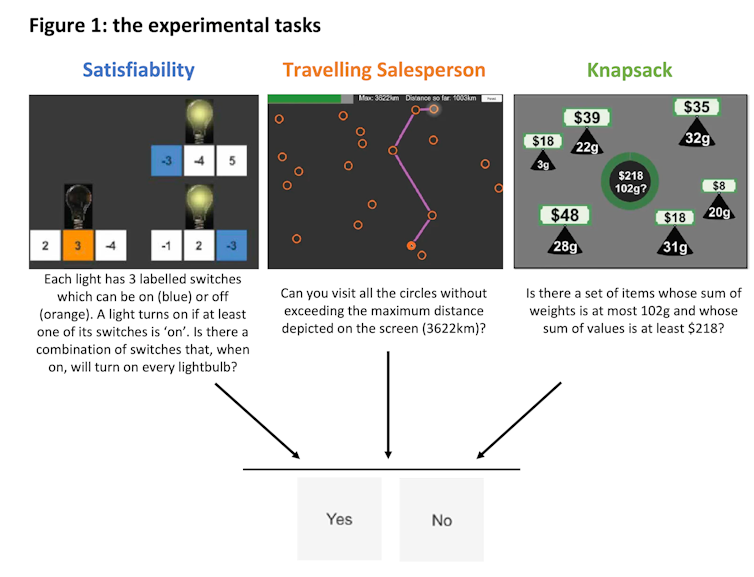
Juan Pablo Franco Ulloa/Karlo Doroc/Nitin Yadav
Each task type requires a different ability to perform well in. Specifically:
- “satisfiability” tasks require abstract logic
- “travelling salesperson” tasks require spatial navigation skills and
- “knapsack” tasks require arithmetic.
All three are ubiquitous in real life and reflect day-to-day problems such as software testing (satisfiability), planning a road trip (travelling salesperson), and shopping or investing (knapsack).
We recruited 67 people, split them into three groups, and made each group solve between 64-72 different variations of one of the three types of task.
We also used computational complexity theory and computer algorithms to figure out which tasks were “high complexity” for a computer, before comparing these with the results from our human problem solvers.
We expected – assuming computational complexity theory is congruent with how real people solve problems – that our participants would spend more time on tasks identified as being “high complexity” for a computer. We also expected lower problem-solving accuracy on these tasks.
In both cases that’s exactly what we found. On average, people did twice as well on the lowest complexity cases compared to the highest complexity cases.
Computer science can measure ‘complexity’ for humans
Our results suggest effort alone is not enough to ensure someone does well on a complex problem. Some problems will be hard no matter what – and these are the spaces in which advanced decision aids and AI can shine.
In practical terms, being able to gauge the complexity of a wide range of tasks could help provide people with the necessary support they need to tackle these tasks day-to-day.
The most important result was that our computational complexity theory-based predictions about which tasks humans would find harder were consistent across all three types of task – despite each requiring different abilities to solve.
Moreover, if we can predict how hard humans will find tasks within these three problems, then it should be able to do the same for the more than 3,000 other NP-complete problems.
These include similarly common hurdles such as task scheduling, shopping, circuit design and gameplay.
Now, to put research into practice
While our results are exciting, there’s still a long way to go. For one, our research used quick and abstract tasks in a controlled laboratory environment. These tasks can model real-life choices, but they’re not representative of actual real-life choices.
The next step is to apply similar techniques to tasks that more closely resemble real-life choices. For instance, can we use computational complexity theory to measure the complexity of choosing between different credit cards?
Progress in this space could help us unlock new ways to aid people in making better choices, every day, across various facets of life.
Karlo Doroc, PhD Candidate in Decision Science, Centre for Brain, Mind and Markets, The University of Melbourne
This article is republished from The Conversation under a Creative Commons license. Read the original article.